The varying definitions of the term 'social
network' reflect its conceptual and
methodological development initially in
mathematics (graph theory) and sociology, and
more recently in environmental sustainability and
related interdisciplinary areas, particularly
resilience of social-ecological systems.
Network research focuses on human or
organizational actors and their social relationships,
and connections among them. For the purposes
here, 'social network' refers to institutional actors
and their linkages, as well as other actors
(individuals, organizations, interest groups etc.). It
relates to the analysis of governance and decisionmaking
networks, which are close to the concepts
of policy and governance networks (e.g. Blanco et
al. 2011). By including multiple types of actors it
recognizes that informal ties as well as formal ones
are deeply involved in 'governance' (e.g. see
Pelling et al. 2008).
A number of methods are emerging that can
identify the various actors (or stakeholders)
involved in decision processes, and map out
these linkages. These can be represented
(visually) and analysed with network maps. These
can be further analysed, in qualitative or
quantitative terms using social network analysis
(for a summary see Taylor et al., 2012). The
background and key benefits of the approach are
provided in Box 1. Participatory social network
mapping and analysis reveals insights about the
substance of these relationships by making
explicit the types of flows between actors (e.g.
information, money, advice, policy, etc.) and the
perceptions of influence and power in the
network. Quantitative SNA provides a variety of
measures/indicators to help describe the overall
relational structure of a social network, as well as
the roles of individuals within it.
SNA can provide insights which can then be
explored further with other methods, notably
follow-up interviews, statistical analysis, agentbased
modelling and participatory scenario
creation. SNA can be undertaken using
qualitative or quantitative methods. The main
difference is that quantitative SNA graphs are
'whole' networks rather than qualitative egocentric
networks based on the perception of
(usually) just one actor. They are also more
comprehensive (i.e. more nodes and links) and
can be quantitatively analysed with SNA
software using standard statistical tests.
Box
1: Key features of network mapping and social network analysisAdapting problem framings. The
initial visualization of a stakeholder-knowledge network can provide
areas for further exploration and research, e.g. identifying malleable
barriers (Moser & Ekstrom, 2010) or informal networks and
‘shadow spaces’ (Pelling et al. 2008), as well as
‘bridges’, ‘boundary-spanners’
(Berkes and Folke, 1998) and different types of
‘flows’ of resources including ‘informal
capital’. These can be highly significant in facilitating
change and influencing policy processes, even if intangible in nature.
It is quite common to find ‘discourse coalitions’
with a shared understanding of the problem, but not necessarily the
same ‘world-view’, or ‘advocacy
networks’ where the ‘world-view’ may be
the same but approaches differ (Turnpenny et al., 2005). Social network
analysis can help understand how and why actors behave the way they do,
through analysis of the structural pattern of relations (topology). It
provides valuable insights to problem framings and how uncertainty is
dealt with. These characteristics help in climate adaptation
‘problem framing’ and understanding different
decision-making regimes.
Facilitating collaboration. Social
processes express the structural pattern of relations in networks and
show how outcome variables influence how networks change and evolve
over time (Borgatti and Foster 2003). The existence of subgroups or
clusters can affect the level of cohesion. For example, weak ties can
have negative effects on the capacity of subgroups to collaborate. The
issue of temporal as well as spatial scales is significant, since the
time horizon for decision lifetimes amongst actors can act as a barrier
(UK CCRA, 2012). Working cooperatively and collaboratively across a
network appears to be an effective way of creating change. Single
organizations can access (and benefit from) the depth and breadth of
resources but also the knowledge, understanding, skills and expertise
needed to build adaptive capacity. Such work is challenging to
coordinate, requiring skill and resources, which can be provided by a
‘Linking Pin’ organization (Carley and Christie,
2000), i.e. for cross-organizational support. Network mapping can
identify areas where these changes can occur and the discussion and
analysis of conflicting or synergistic goals (barriers to cooperation
and collaboration). Identifying these goals is also part of the
participatory process when creating network maps. Not all flows are
‘positive’. Bodin and Crona (2009) cite examples of
the correlation between network density and joint action. They also
note that there may be a threshold above which network density becomes
counter-productive in facilitating collective action (e.g. Oh et al.,
2004 in Bodin and Crona, 2009) due to the homogenization of information
and a lack of ‘new’ knowledge leading to less
efficient resource use and/or reduced capacity to adapt to changing
conditions.
Agents
of change. Network topologies can be analyzed at the
network-level, but also at the node-level focusing on institutions or
actors. Assessing the position of the actor in the network and the
number and strength of their relationships reveals their structural
position to influence other actors. The centrality of an actor allows
analysis of the level of influence, but also the role they can play in
the network as a bridge that connects others (Cash et al. 2002). An
actor connecting with many others has the ability to influence the
flows between actors. Identifying central actors is a useful way to
understand dominant decision framings, how these are used and the
effect on collective action. In this regard, central actors located in
strategic positions can be potential ‘agents of
change’ in the network or ‘adaptation
champions’.
Inter-agency coordination. Options
identified by different parts of a governance system often relate to
who has control over the decision process, jurisdiction, political
interests, funding, etc. (Renn, 2008 in Moser and Ekstrom, 2010). If
the breadth of the system of concern covers many jurisdictions, the
issue requires cross-coordination to implement options (Moser &
Ekstrom 2010). The beneficial aspect of clusters is that they may
facilitate the development of specialized and tacit knowledge within
their own sub-groups. This is valuable for the knowledge diversity of
network as a whole, provided that there are also mechanisms for
knowledge transfer and boundary-spanning (Berkes and Folke, 1998) to
facilitate ‘joined-up thinking’ between
specializations, to lead to new knowledge and action. This can enhance
integrated management and cross-sectoral planning. Without knowledge
transfer, the opposite effect can manifest itself – very low
collaboration and cooperation or reconciliation of actors with
differing goals and objectives.
Types of Networks. A
simple illustration of types of network topologies is shown below
(Figure 1). These examples outline different types of networks based on
number of peer connections, density of relations, role of boundary
nodes between isolated networks and degree of cohesiveness, inheritance
of links as organizational structures, subgroups interconnectivity and
degree of network centralization.
Type 1. Individual
action predominates. While people are connected in various ways, most
actions are at the organisation/individuals own level and independent
of what others believe or are doing. In this type of network, the
psychology of individual action dominates. At this level, there may be
a diversity of approaches to uncertainty and there is little need for a
consensus view. The construction of the problem is usually highly
constrained and mostly short-term with rather limited information on
long-term futures.
Type 2. Individuals
and groups are connected in an egalitarian space. There are various
links but the network tends to be ‘like-minded’ and
the structure of the problem is similar across actors. Uncertainty may
not be explicit—rather reduced to tacit assumptions common in
peer networks and reflected in cultural and group norms rather than a
science-policy dialogue as such.
Type 3. Many
organizations have hierarchical decision making with a leader (and even
an meta-level organisation e.g. a Board) defining policy that is
translated into strategy and action. Uncertainty can be explicit,
although it tends to be wrapped into how the organization is structured
and procedures that are in place for other purposes. Co-management
would be the opposite to this, where multiple actors are involved in
the governance to varying degrees as opposed to top-down centralized
management. Adaptive co-management emphasizes flexible joint management
processes, which will allow the continuous application of new knowledge
where relevant (Bodin and Crona, 2009).
Type 4. A hybrid of
two or more kinds of networks, which is often the reality. Two
egalitarian networks for instance might be linked, each with its own
approach to uncertainty. In such cases, there is more than one decision
framing in play and uncertainty may enter the decision in different
ways.
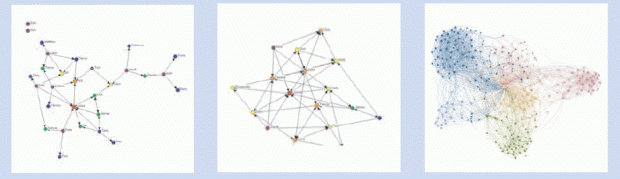
Figure 1.
Illustrations of peer-oriented network types.
Type
1 (left): Individualistic, few links between nodes.
Type 2
(centre): Egalitarian more connected.
Type 4 (right):
Multiple networks in a hybridisation.
There
are many ways to apply SNA to a particular context. The main steps in
quantitative and qualitative SNA are outlined below.
Quantitative Social Network
AnalysisQuantitative
SNA aims at capturing the entire relevant network. The steps for
quantitative social network analysis are:
- Clarifying
objectives and defining the scope of analysis (e.g. mapping a knowledge
domain).
- Developing a survey methodology and
designing the questionnaire.
- Identifying the
participants (network) and providing justification for boundaries (if
appropriate).
- Collecting survey data and gathering
further information from other resources.
- Analysing
the data through formal methods.
- Reviewing process
and outcomes to identify problems/opportunities.
- Designing
and implementing actions to bring about desired changes.
- Mapping
the network again after an appropriate period of time.
This
is a resource intensive task, and field research requires very high
response rates, and high resources, as any missing data can weaken the
analysis. Other approaches using existing data (e.g. co-citation
networks, online databases, household surveys) can also be considered,
though it is not always easy to extract relational information or
perform suitable data transformations.
Qualitative Social Network
AnalysisQualitative social network
analysis or social network mapping (SNM) takes advantage of the early
steps above – the interviews, surveys or focus group
discussions – to elicit information on the relevant networks.
It can facilitate rich discussions, shared understanding and increased
awareness between different stakeholders. This can be part of a rapid
appraisal before detailed analysis begins. It can also identify entry
points for policy influence (Turnpenny et al., 2005) and other
‘flows’ of resources which can include
‘informal capital’.
A number
of approaches and tools can be used for network analysis. Following
Schiffer (2010), the NetMap guidance is a useful example for applying
the approach in a participatory way. The method is usually applied
using flipcharts, post-it notes and flat counters with a group of
stakeholders who are split into homogeneous groups related to the type
of institution they belong to e.g. Government level representatives,
NGOs, farmers, etc.
Once the adaptation research
question is well defined, participants go through the mapping exercise
including an analysis of the network, and then come back into plenary
for a discussion of the different networks from the different
stakeholder perspectives. This enables a better ‘shared
understanding’ of differing world views.
A
range of software exists for both quantitative and qualitative SNA.
This includes software for visualisation and analysis, such as GEPHI,
UCINET, ORA and NetDraw.