Robust Decision Making (RDM) is a decision
support tool that can be used in situations of deep uncertainty. RDM is
premised on the concept of “robustness” rather than
“optimality”. The approach was developed to help
policymakers make more informed near-term decisions which have
long-term consequences.
RDM involves testing
near-term strategies across a large number of plausible future states.
The primary aim is to help policymakers anticipate or mitigate the
negative impacts of possible future surprises resulting from the
interaction of factors (exogenous uncertainties) outside of their
control, with measures that are within their control. This is described
as decision making under situations of deep uncertainty, i.e. when
little or no probabilistic information is available.
The
approach can be applied when traditional risk information (e.g.
well-defined probability distributions) is not available, when there is
no agreement on the conceptual models to use, or how to evaluate the
desirability of alternative outcomes. RDM aims to help decision makers
to take robust or resilient decisions today, despite imperfect and
uncertain information about the future.
RDM has
developed as an analytic, scenariobased approach for strategic
decision-making. The formal application of the approach involves the
combination of both qualitative and quantitative information through a
human and computer-guided modelling interface (Lempert et al, 2003:
Groves and Lempert, 2007). This powerful combination surpasses the
analytical power of traditional qualitative and quantitative decision
support tools. This computer based analysis allows RDM to evaluate how
different strategies perform under large ensembles, often of thousands
or millions of runs, which reflect different plausible future
conditions (Lempert et al, 2003). Iterative and interactive techniques
are then applied to “stress test” different
strategies, identifying potential vulnerabilities or weaknesses of
proposed approaches (Dessai et al., 2009). The formal application of
the approach is characterised by the use of data mining algorithms
which carry out vulnerability-and-response-option analysis (Groves and
Lempert, 2007).
The formal application of RDM
involves a series of steps, set out in Figure 1 below (Groves et al.,
2008). RDM analysis begins by structuring the problem, but instead of
characterising key uncertainties (or more accurately, risks) as a
prelude to optimally ranking strategies, the analysis proposes
alternative strategies. Uncertainties associated with the parameters
defining these strategies are then characterised, assigning a range of
uncertainty values for each variable using stakeholder consultation or
other approaches.
Each strategy is then assessed
over a wide range of (computer-generated) scenario futures.
Combinations of uncertainty parameters that are most important to the
choice between strategies are statistically derived and a summary of
key trade-offs among promising strategies developed (Groves et al,
2008).
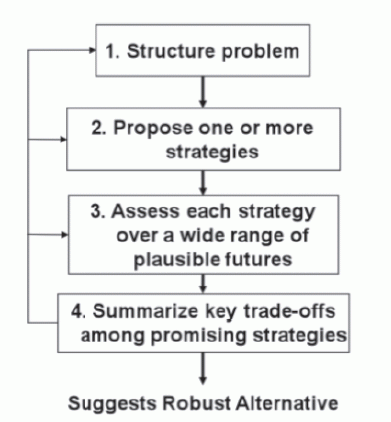
Figure 1. The RDM
processSource: Groves et al., 2008The
analysis of strategies across the scenario futures is measured with
performance measures, which are used to measure pre-selected, desirable
outcomes. Ideally, an RDM analysis helps to identify a robust strategy
– one that performs well over a very wide range of scenario
futures. In the event that a robust, well performing strategy
is not identified, the iterative process of strategy reformulation
begins again with stakeholders.
The formal
application of RDM tests many strategies against computer-simulated
future scenarios, considering exogenous factors (outside the decision
maker’s control) and policies or options (within their
control), linking these with functional relationships, and assessing
strategies against the performance measures in quantitative terms. The
former method involves application of statistical or datamining
algorithms.