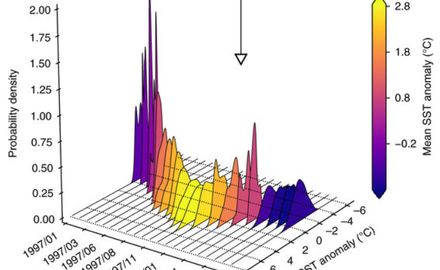
“We view time series not as a measurement sequence over time but rather as a sequence of probabilities over time,” says Bedartha Goswami from the Potsdam Institute for Climate Impact Research (PIK), lead-author of the study now published in Nature Communications. “We shift from knowing the value of an observable at a given time to knowing how likely was the observable to have a certain value at that time.”
The team of scientists applied this mathematical method to real-world problems. “Our analysis showed that the transitions identified in daily stock index data for the period 2004-2016 relate to the mortgage crisis, the Eurozone crisis and the Brexit crisis of UK deciding to leave the EU,” says Goswami. “This is certainly not very surprising, but it demonstrated that our concept was working rather well. New insights were gained by applying our method to the sea-surface temperatures from the Pacific. We found that the transitions in the El Niño are more likely when two kinds of temperature oscillations are in a similar phase, which opens up a new aspect to our of understanding of the El Niño – a phenomenon which influences the weather around the globe.”
“This is a major step forward for analyzing dynamical systems in general – not just the climate, but also for instance in economics,” says co-author Jürgen Kurths, head of PIK’s research domain Transdisciplinary Concepts and Methods. “We extend the framework of recurrence analysis, a tool to investigate features such as memory or disorder from patterns encoded in the return characteristics of a given state.” This new approach is particularly important for identifying abrupt transitions in the Earth’s past climate, such as detecting dips in the Asian Summer Monsoon in the past 10.000 years.
Article: Bedartha Goswami, Niklas Boers, Aljoscha Rheinwalt, Norbert Marwan, Jobst Heitzig, Sebastian F.M. Breitenbach, Jürgen Kurths (2018): Abrupt transitions in time series with uncertainties. Nature Communications. [DOI:10.1038/s41467-017-02456-6]
Weblink to the article: https://www.nature.com/articles/s41467-017-02456-6